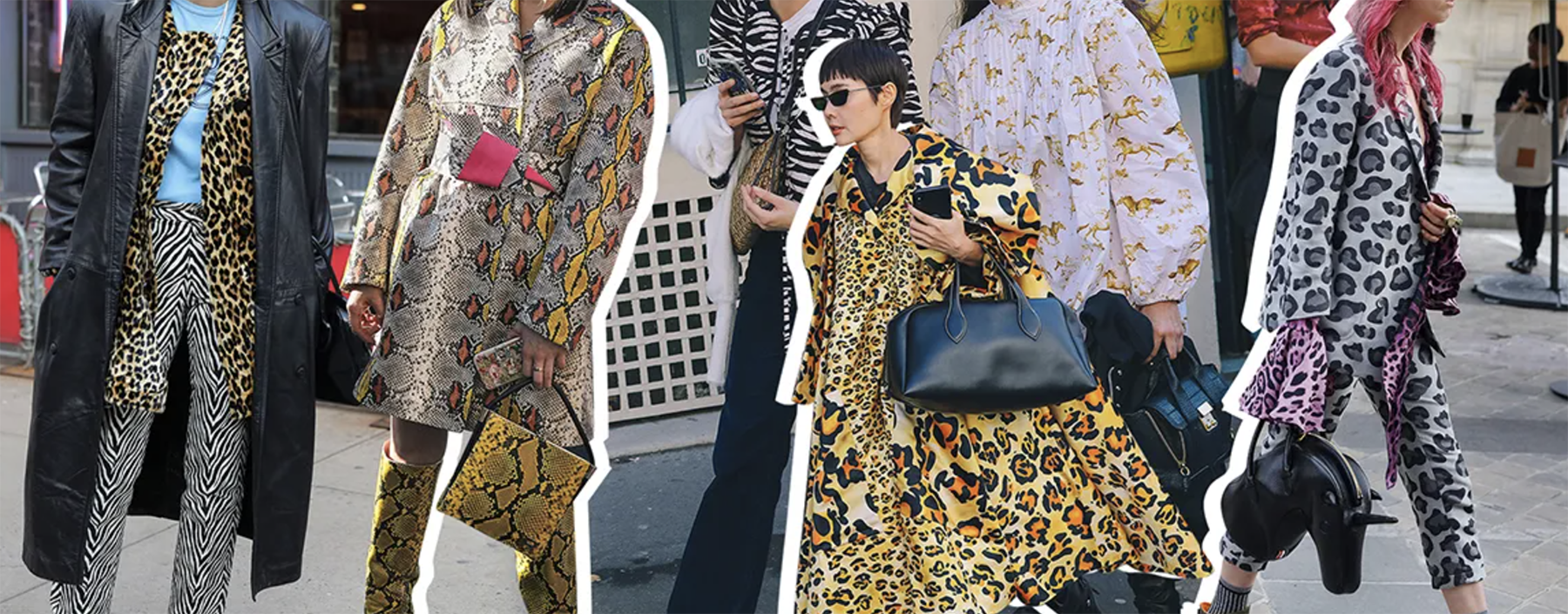
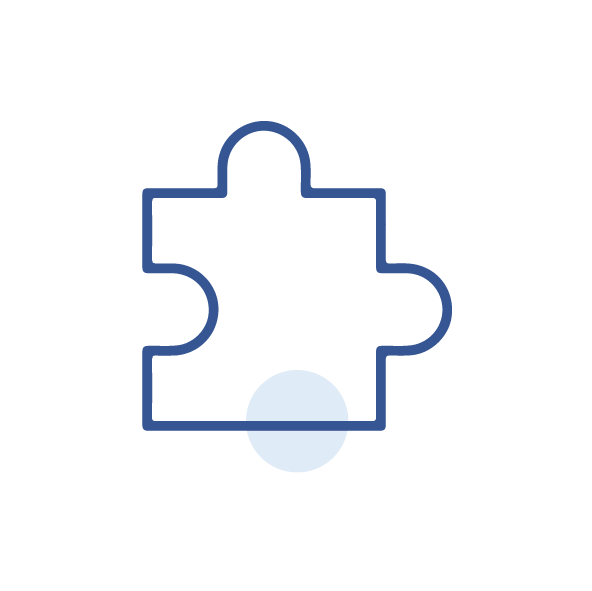
CHALLENGE
Product search is arguably the most influential touchpoint in a customer's shopping process
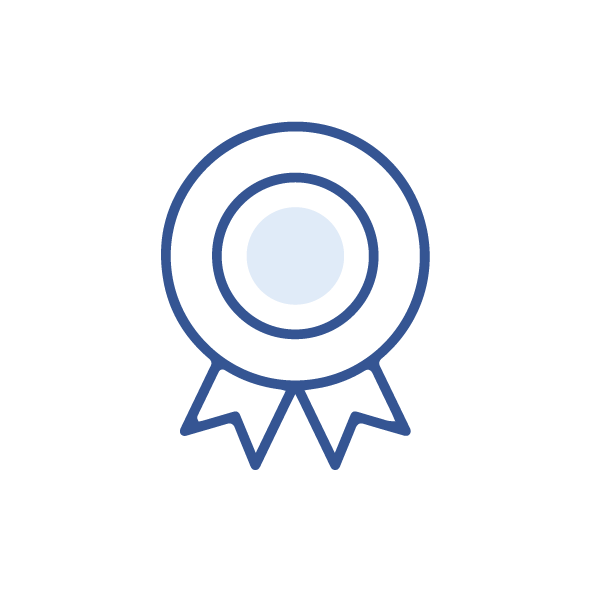
RESULTS
Our classifiers are built for all variations of print with accuracies of 80-85%
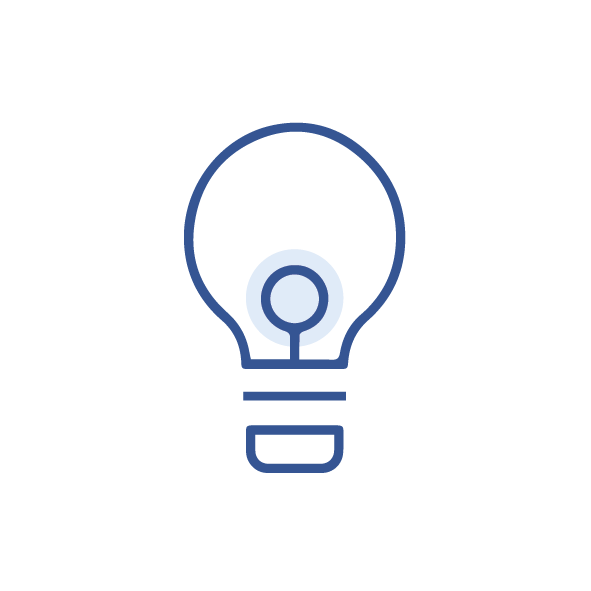
SOLUTION
Shopper has to decide tags like print and pattern by trial and error
Is it possible for ML models to enhance product discovery with accurate print and pattern suggestions
Print and pattern differ so little that it's almost impossible to tell them apart. The terms are often used interchangeably. In fact, a print is a pattern. We’ll give you a second to think about that.
Now, imagine training an AutoML model to understand the same. While it wasn't easy, we'll tell you why it was worthwhile. Imagine if a customer looked up floral tops and the results were a mix of tropical and abstract prints. This could affect the customer experience and potentially result in the loss of a customer.
Product search is arguably the most influential touchpoint in a customer's shopping process, which is why retailers must make it seamless. And we're here to help with that.
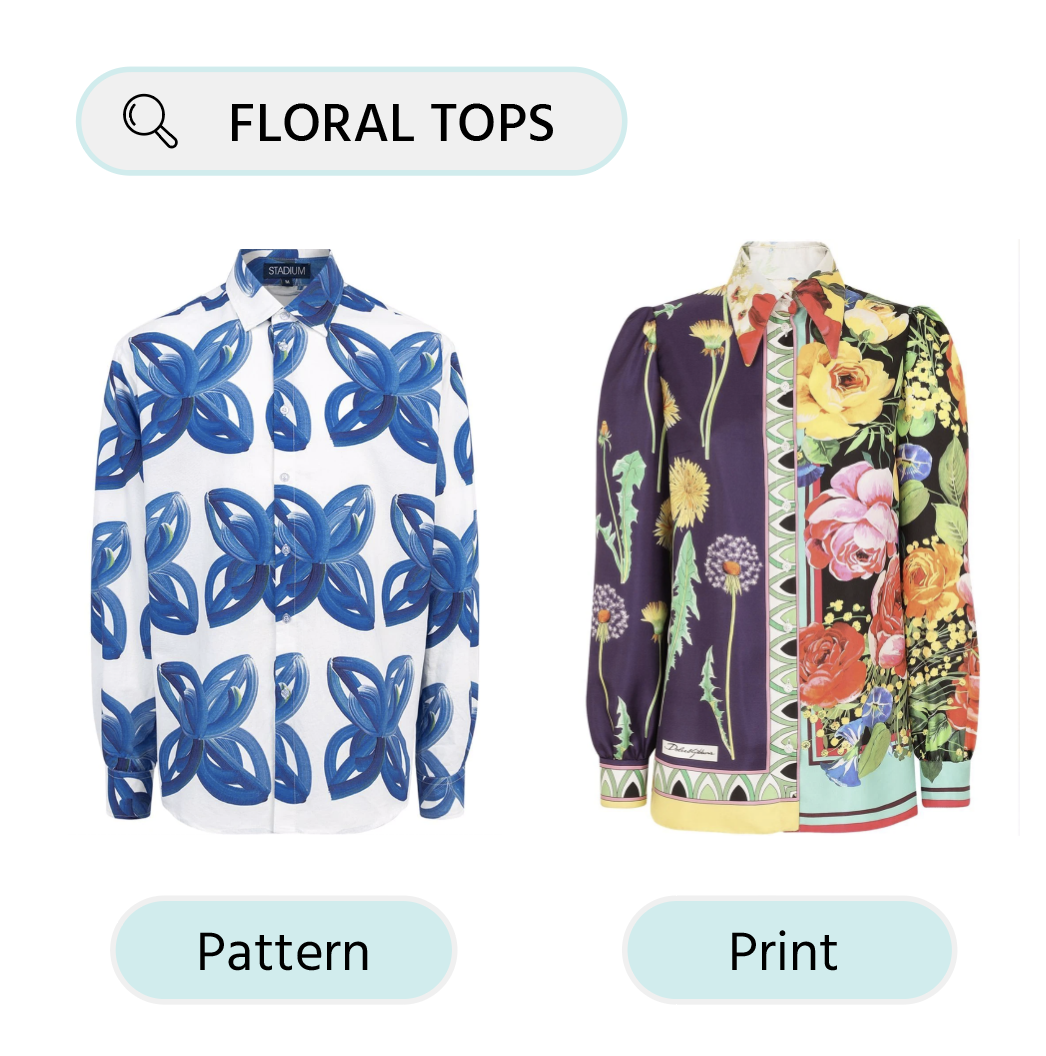
CURRENT SOLUTION
Brands vary in how they tag prints and patterns. There is no universal taxonomy. So, it is left to the shopper to decide by trial and error.
HOW AI STUDIO HAS SOLVED IT
The first step was to train the model to understand the diverse prints and patterns in clothing. To do this, a certain set of training images was required for each label of every attribute.
Let us simplify that for you. An attribute is a specific feature of the garment such as Print, whereas labels are the values of the attribute: Floral, checks, conversational, etc. We had to create a taxonomy that identified every variable to prevent any kind of misinterpretation.
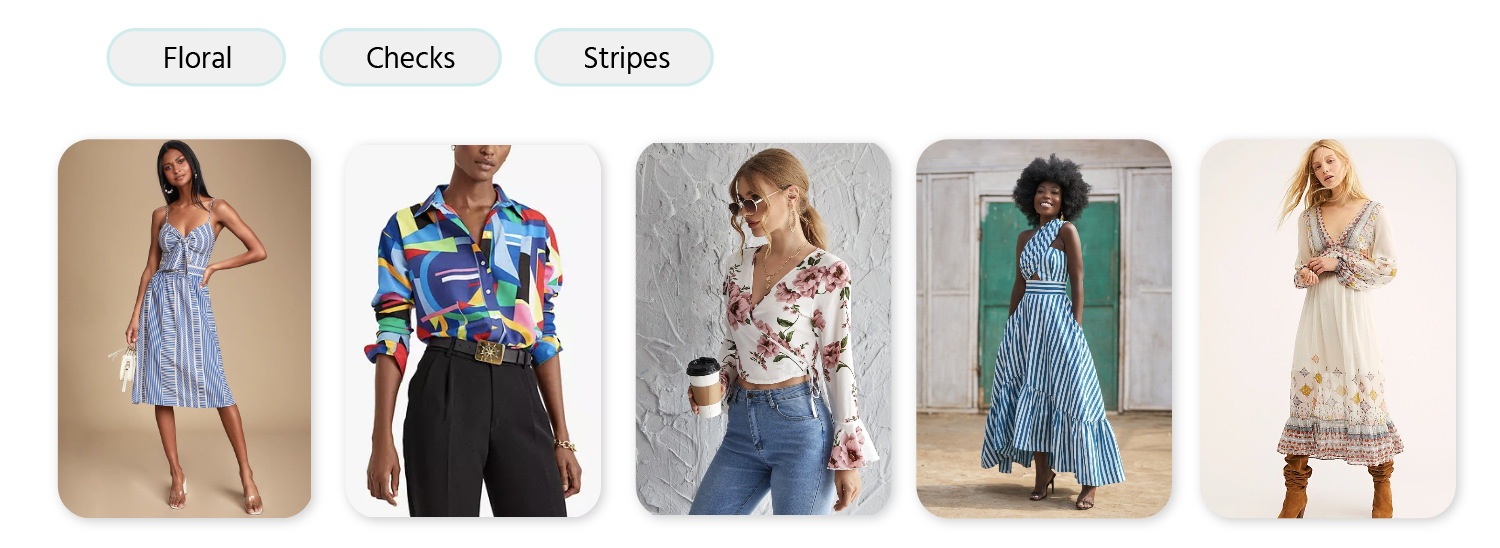
Solid, animal, stripes, color-block, graphic, branding, camo, abstract, polka, etc were the various values under the Attribute Print.
Let’s circle back to the images required for data collection. Our collection of eCommerce images included 80% images with plain backgrounds and some that were crowdsourced. We also added 20% of street images with noisy backgrounds. This bias towards eCommerce images was intentional as that was the final use case.
Any algorithm is only as good as the data used to build it. This project was especially demanding as the model was trained to recognize something as complex as print. Having collected sufficient data, we split the data set into training and test data. The process is an iterative one, data is consistently fed into the ML models.
Accordingly, the accuracy of the generated data was over 85%. The data was further enhanced by manual quality control. There you have it, an AutoML model that generates images with accurate print and pattern tags.
Some insightful suggestions from the model include:
1. The auto-removal of duplicate images reduces clutter.
2. Insights on failed images and the wrongly predicted value. This can be checked and corrected manually, thereby improving accuracy.
3. Confusion Matrix is a numerical table that shows the wrongly predicted labels in classifier training, enabling you to further improve the quality of product generation.
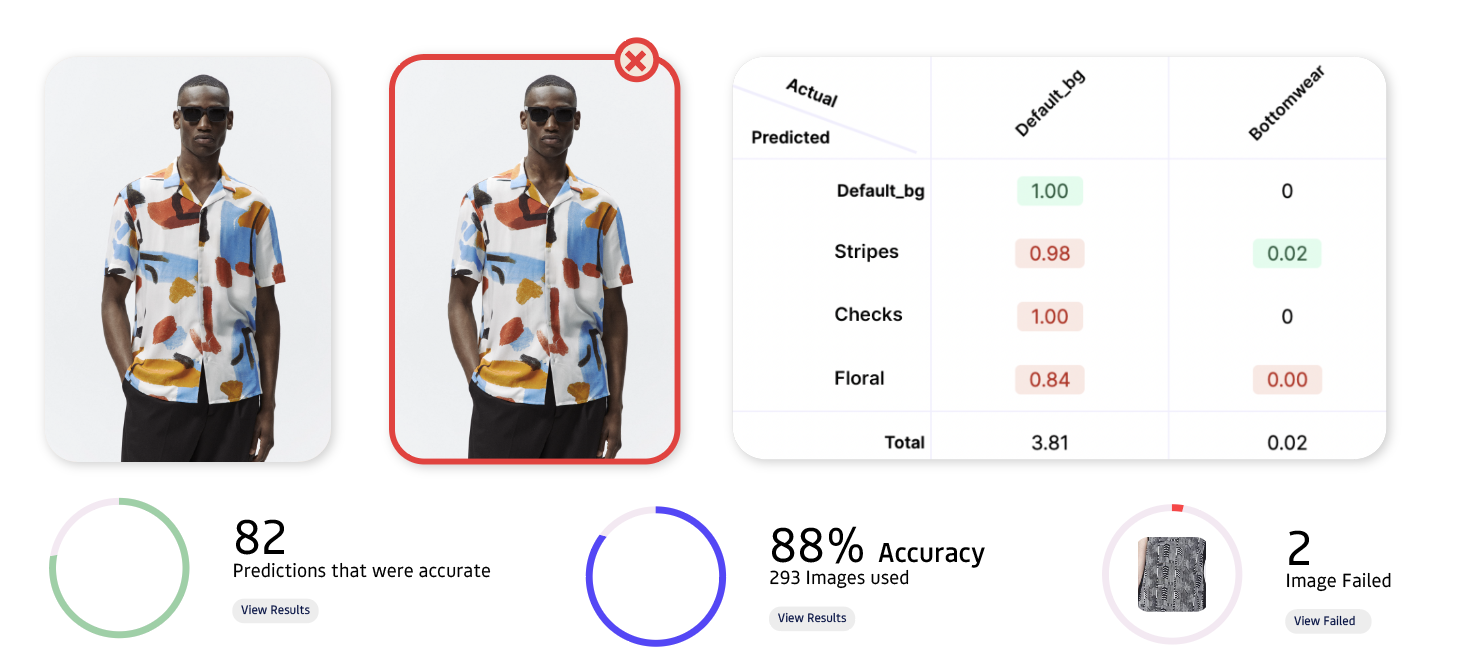
BUSINESS IMPACT
Our classifiers are built for all variations of print with accuracies of 80-85% which can be further enhanced for a particular dataset. This reduces our manual QC effort during the cataloging process.
Contact us to book a free demo.
MODEL BUILDER: Shruthi – Fashion Analyst and Classifiers Team.