
The Problem With Visual Search In Fashion… And How To Fix It
How often has this happened to you?
You love browsing shopping apps and are always on the lookout for interesting clothing that others are wearing. You’ve heard all about “visual search” that retailers now offer in their apps, so you open the camera and upload a picture to find similar matches.
You’re disappointed with the suggestions though…
Successful fashion retailers are really starting to adopt innovative technology for their customers, and this increasingly relies on AI. To retain their edge in an extremely competitive space most retailers are exploring “visual search” as a starting point. It’s easy to understand why -
- Pinterest (whose users overlap significantly with fashion retailers) reported 600 million visual searches in 2018 on its platform with fashion being the most popular category. (https://newsroom.pinterest.com/en/post/celebrating-one-year-of-pinterest-lens)
- Gartner predicted in 2017 that visual and voice search would increase eCommerce revenue by over 30% by 2021.
While there are many successful case studies presented for visual search, most retailers suggest adoption levels are much lower than expected. Hence, the success stories we see are based on a small set of users and because of this visual search is not even close to contributing to eCommerce revenue the way Gartner predicted.
We at Streamoid have been working hard to understand why this is and address it. So what did we find? How can visual search be perfected to ensure that you have a great experience next time you use it?
Why are users frequently disappointed with the results?
- Sometimes, the results are not from the category that the user is looking for.
- The results are not visually similar at all
- The results (although relevant) don’t have the aspect the user was looking for, like a pattern
Why does this happen?
- Not every retailer has a large enough catalog to find an exact or even close match to what the user has uploaded
- Not all users like the same features of a garment, some may be searching for the pattern, while others the cut.
To fix this, Streamoid has adapted visual search, by giving retailers a framework that allows them to make changes to the matching criteria. This means that certain features of a suggested item are taken into account more.
How have we done this?
- By creating a Universal Fashion Ontology that spans 50+ attributes and contains 2000+ visual features, Streamoid is able to capture detailed information about a garment just from the image.
- A retailer’s catalog goes through our auto-tagging system (Autoscribe) which tags garment information at a far more granular level than any retailer could hope to do manually.
- Retailers then have the flexibility to decide the visual matching criteria for every category. We recommend fewer criteria for retailers with smaller catalogs so there’s a higher chance of showing results.
- Our Superintelligent platform already understands the fashion world and curates default recommendations for attributes that should be matched in each category.
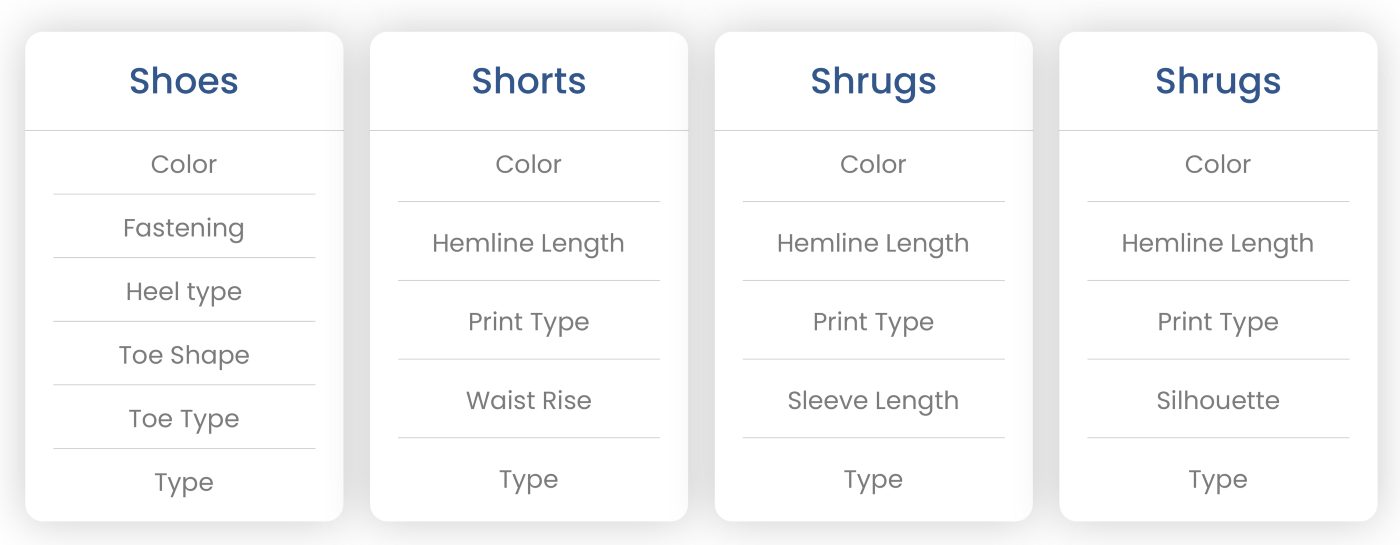

Retailers can take this experience even further by giving users the choice to decide which aspects they love, keeping the user engaged even if the initial results weren’t exactly what they wanted.
The same user can now get a meaningful visual search experience across different retailers regardless of catalog size.

On a smaller catalog, the users can search for example just for matching prints.
On a larger catalog, the users can find an exact match for the product they’re looking for.


Importance of Product Taxonomy: Role of AI in Automating & Improving Taxonomies
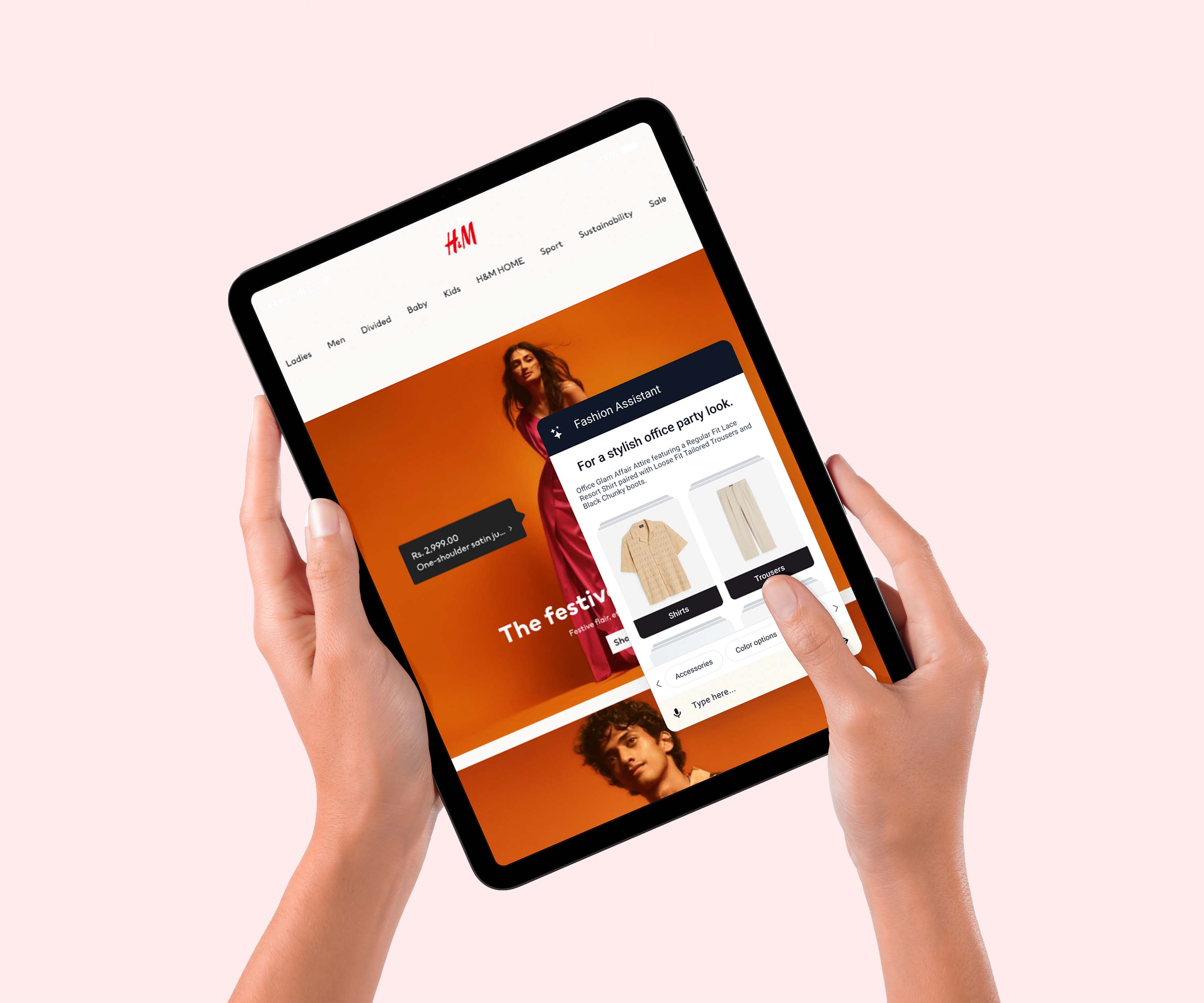